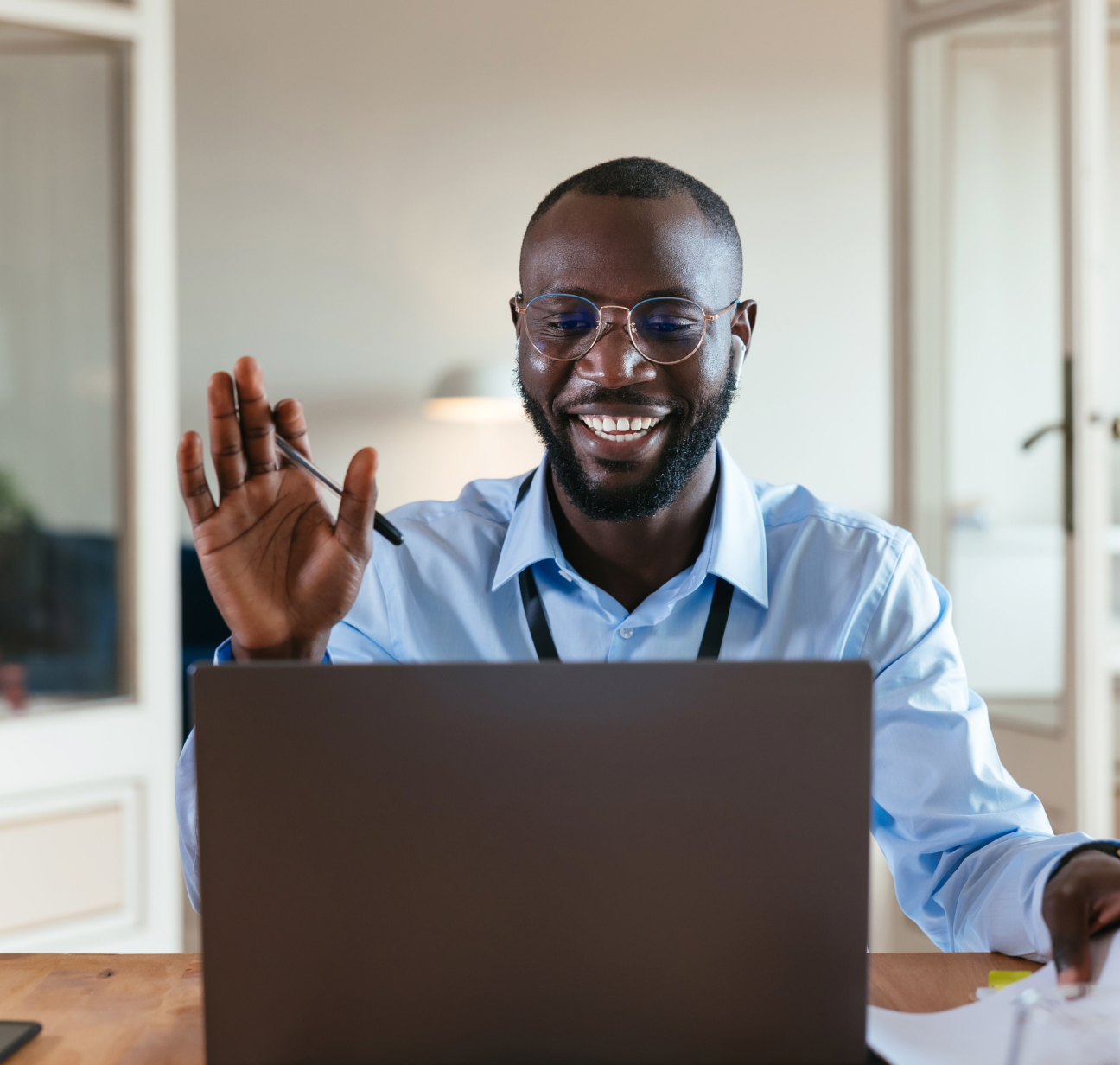
Learn how to maximize your data and minimize your efforts with FME
Join our free and interactive workshop to learn how to make the most use out of your data with FME. The FME Accelerator will show you the basics of how to automate your data integration workflows and connect to 450+ formats.
Non-profit, teacher or student?
We have Free Licenses available to help jump start careers or support your learning, research and charity.
See If You Qualify
Questions about the platform?
We love questions, discussions, and providing demos to help you get started on or to continue your data integration journey.
Contact Us
Join the FME Community
Our growing community has a wealth of FME knowledge with over 20,000 active members worldwide, where you’ll find everything from support to training.
Explore the Community